
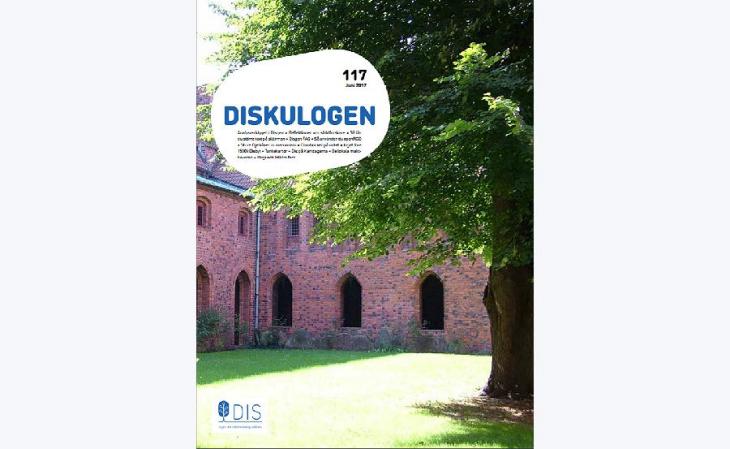
Cc Vision More Growth Within The Last Year World Of Print. Ccvision Car Special V20 Divas Unlimited Inc. 1051- solidcam … 117- Coade Caesar II 5 … 483- CC Vision Car Spec 2008 v14. 40- Fs2004 – airplane – Ariane Boeing 737-300 Easyjet Special Ed … 1043- ProgeSoft ProgeCAD 2008 Professional v8.0.22.18. Led Zeppelin … sonnenfreunde sonderheft 117 20.30- Digital DATCOM 40- Fs2004 – airplane – Ariane Boeing 737-300 Easyjet Special Ed … 117- Coade Caesar II 5 463- COADE … 419- Bentley ProSteel 3D v18.0 929- Bentley … 483- CC Vision Car Spec 2008 v14 308- TLF … ccvision car special crackĬcvision Car Special V18 Download Torrent Download Torrent Download ccvision car specialĬcvision car special, ccvision car-special vector drawings, ccvision car special crack, ccvision car special downloadĬcvision Car Special V18 117 ccvision car special, ccvision car-special vector drawings, ccvision car special v19, ccvision car special …. It is possible that your Scanner driver ccvision software download is not compatible with the newer version of Windows.
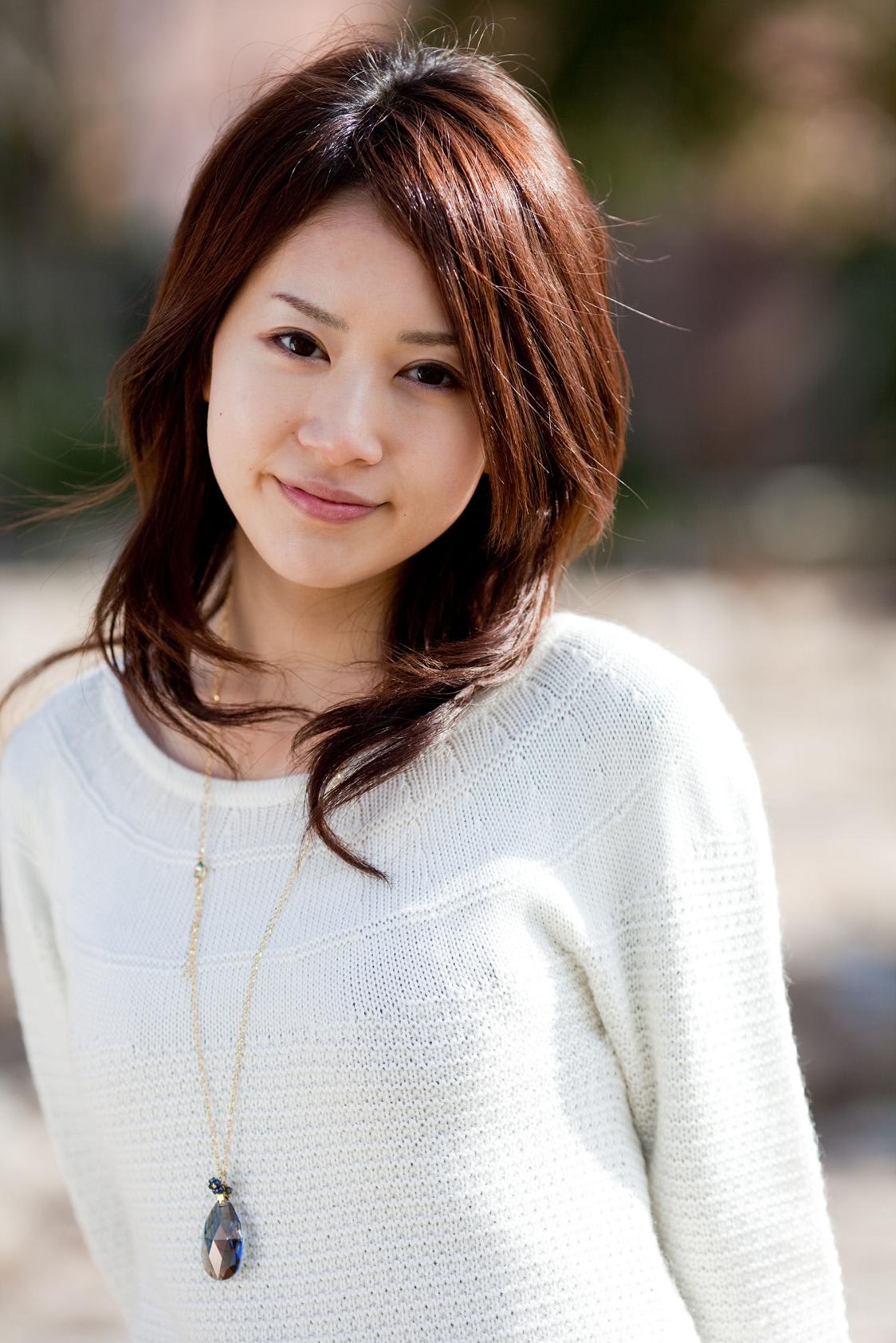
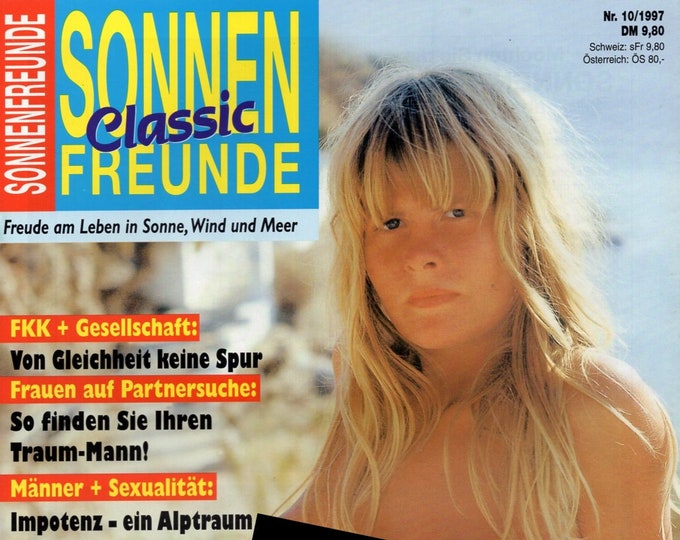
3.0.006 … Cult3D Designer v5.3.0.117 … CCVISION CAR DECO 1 Ai for Illustrator. Principal Component AnalysisPrincipal Component Analysis, or PCA for short, is a method for reducing the dimensionality of data.It can be thought of as a projection method where data with m-columns (features) is projected into a subspace with m or fewer columns, whilst retaining the essence of the original data.The PCA method can be described and implemented using the tools of linear algebra.PCA is an operation applied to a dataset, represented by an n x m matrix A that results in a projection of A which we will call B.Ccvision Car Special V18 117 > DOWNLOAD
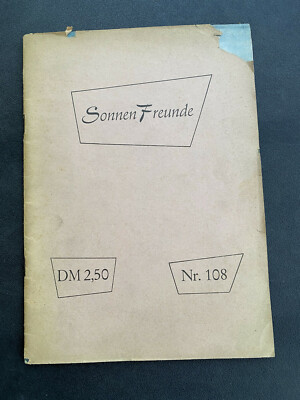
Let’s walk through the steps of this operation. Values, vectors = eig(V)The eigenvectors represent the directions or components for the reduced subspace of B, whereas the eigenvalues represent the magnitudes for the directions. For more on this topic, see the post.The eigenvectors can be sorted by the eigenvalues in descending order to provide a ranking of the components or axes of the new subspace for A.If all eigenvalues have a similar value, then we know that the existing representation may already be reasonably compressed or dense and that the projection may offer little.
